AI, zBlog
Unlocking AI Operationalization with Next-Gen Machine Learning Ops
trantorindia | Updated: September 20, 2021
Five-year-old AlphaGo and the relatively recent AlphaFold are two of the seemingly futuristic applications of Machine Learning. The former is a program trained to play chess that defeated Gary Kasparov, the then World Champion. In contrast, the former helps generate proteins and aids in solving one of the most challenging problems in the field of Biology. Applications like these have paved the way for an ever-increasing interest among the masses in Machine Learning and Artificial Intelligence concepts.
However, despite this growing interest, 85% of AI projects don’t deliver the intended promises to businesses. These numbers indicate that some gaps need to be filled when it comes to the operationalization of Artificial Intelligence. In 2021, the core challenges that act as a roadblock while working with ML include:
- Access to trusted data
- Multi-cloud strategy
- Automation of machine learning
Luckily, next-generation Machine Learning Operations have the solutions to all of these challenges. Let’s look at that in-depth.
Next-Gen Machine Learning Ops
Next-generation Machine Learning Ops works by tackling all the challenges faced by the traditional methods of working with ML and AI. It includes operationalization, streamlining deployment, and automated execution of ML models. Next-gen MLOps are essentially a standard set of practices that can scale ML to actualize AI’s power. In return, it will allow businesses to deliver machine-led, trusted, and utmost accurate decisions in real-time.
Next-gen MLOps work on the concept of DevOps by merging ML and operationalization. MLOps offers businesses the framework required to cut the clutter from their ML processes and operationalize the ML model development process. Companies mentioned above can set up a continuous delivery cycle of models necessary for fully functional AI-based systems.
By automating ML model development and deployment and offers frameworks to streamline ML operations, next-gen MLOps provides the following benefits to businesses:
- Delivers increased business value of all data projects.
- It makes the data processes and techniques much more efficient.
- Allows ML models to run more accurately with improved results
- Helps businesses achieve more significant revenue and enhanced operational efficiency
- Accelerates digital transformation journey
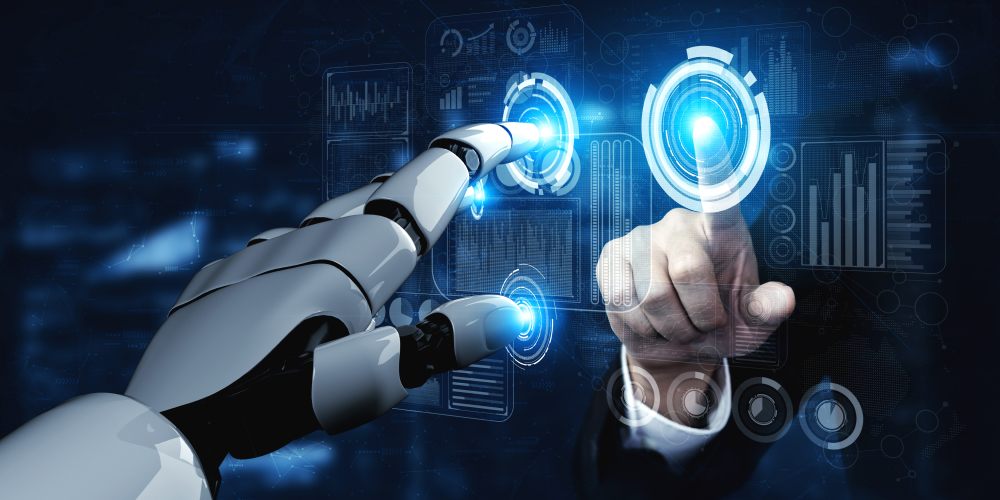
Use Cases of Next-Gen MLOps
Next-gen MLOps aren’t the future – they are already in the present. So far, Machine Learning has helped businesses across different industries to improve their processes and enhance efficiency. However, the traditional approach to ML and AI fails on many fronts. That’s where next-gen MLOps have been offering businesses a breath of fresh air by helping with legacy use cases. Some of the use cases of next-gen MLOps include:
- Banking and Finance: Fraud prevention and detection, customer experience improvement, customer onboarding, algorithmic stock trading, credit scoring, portfolio management, loan processing.
- Retail: Retention forecasting, product usage trends, sales, customer lifetime value, audience segmentation, inventory management, planning next-best action.
- Healthcare: Drug discovery, cancer diagnosis, ICU monitoring, preventive patient care.
- Automotive: Predictive maintenance, improved design, smart energy utilization, quality control, supply chain management.
The above-mentioned use cases are only a few of the many ways MLOps have proved their importance in the business sphere of the future. As you can see, MLOps have helped solve some of the most pressing and legacy challenges of different industries by automating ML deployment and utilizing it to achieve the full potential of AI operationalization.