Digital Transformation, zBlog
What Is Data Annotation: Definition, Types, Tools, Future Trends
atif | Updated: April 26, 2024
Introduction
In the realm of artificial intelligence (AI) and machine learning (ML), data annotation has emerged as a critical component driving the development and deployment of intelligent systems. At its core, data annotation is the process of labeling or enriching raw data with meaningful metadata, enabling machines to extract insights and make informed decisions.
As AI technologies continue to advance and permeate various industries, the need for high-quality, accurately annotated data has become paramount. From self-driving cars to voice assistants and medical image analysis, it plays a crucial role in teaching AI models to recognize patterns, classify objects, and understand context – paving the way for intelligent solutions that can revolutionize businesses and transform lives.
This comprehensive guide delves into the intricacies, definitions, types, tools, and future implications, equipping you with the knowledge to harness the full potential of Artificial Intelligence and drive innovation within your organization.
Understanding Data Annotation: The Foundation of AI Learning
Data annotation is the process of adding descriptive labels, tags, or metadata to raw data, such as images, text, audio, or video. This process transforms unstructured data into structured, labeled datasets that can be used to train machine learning models. Without accurate and consistent data annotation, AI models would struggle to learn and make reliable predictions or decisions.
The annotation process typically involves human annotators, domain experts, or crowdsourcing platforms, who manually label or enrich the data based on predefined guidelines and taxonomies. However, as the volume of data continues to grow exponentially, automated and semi-automated annotation techniques are becoming increasingly prevalent, leveraging machine learning algorithms to expedite the process while maintaining high accuracy.
Types
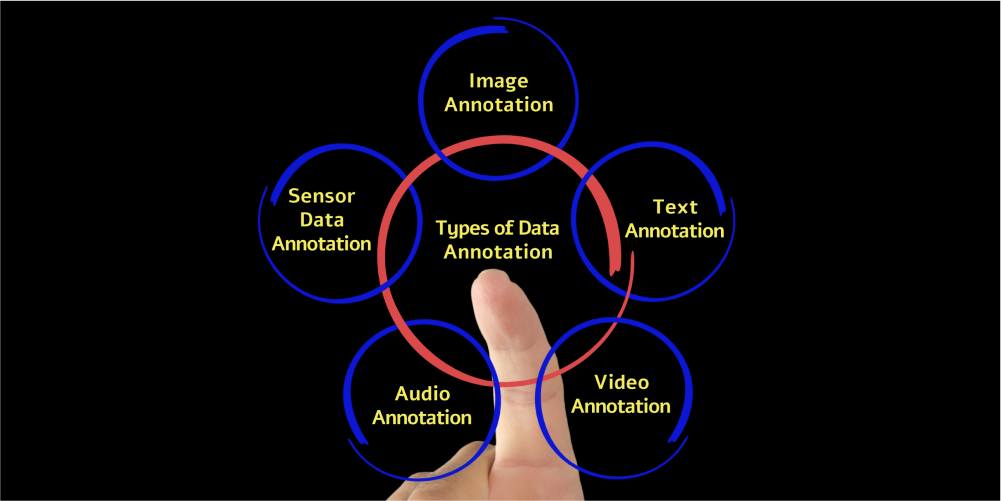
Data annotation encompasses a wide range of techniques and methodologies, tailored to different types of data and specific use cases. Here are some common types:
1. Image Annotation:
- Object detection and localization
- Semantic segmentation
- Image classification
- Instance segmentation
- Keypoint annotation
2. Text Annotation:
- Named entity recognition (NER)
- Part-of-speech (POS) tagging
- Sentiment analysis
- Intent and entity classification
- Relation extraction
3. Video Annotation:
- Object tracking
- Action recognition
- Pose estimation
- Video segmentation
- Event detection
4. Audio Annotation:
- Speech recognition
- Speaker diarization
- Audio event detection
- Emotion recognition
- Transcription and captioning
5. Sensor Data Annotation:
- Anomaly detection
- Time-series forecasting
- Predictive maintenance
- Condition monitoring
These are just a few examples, each tailored to specific AI applications and use cases across various industries, such as healthcare, autonomous vehicles, retail, finance, and beyond.
Tools and Platforms
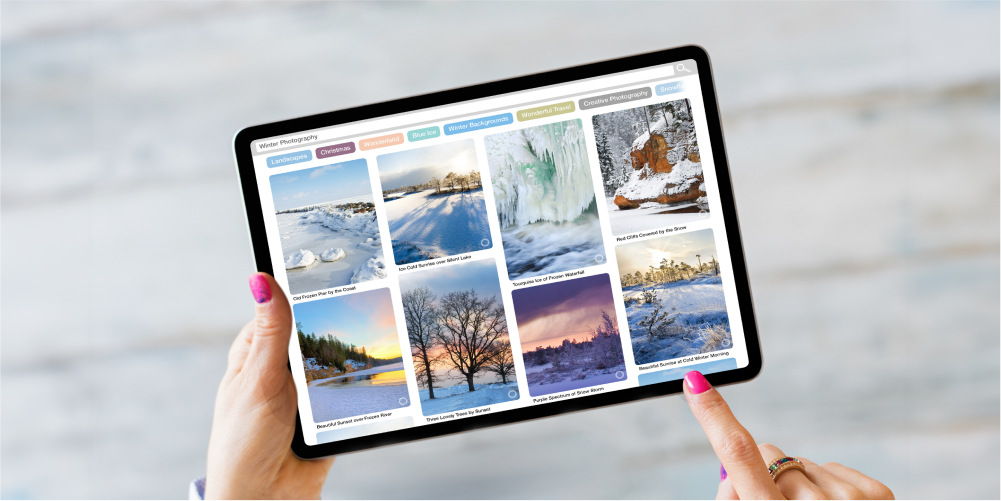
To streamline and enhance the data annotation process, a wide range of tools and platforms have emerged, offering features and capabilities tailored to different types of data and annotation tasks. Here are some popular tools and platforms:
1. Computer Vision Annotation Tools:
- LabelImg
- VGG Image Annotator (VIA)
- RectLabel
- Labelbox
- Roboflow
2. Natural Language Processing (NLP) Annotation Tools:
- BRAT
- doccano
- Prodigy
- Tagtog
- LightTag
3. Audio and Video Annotation Tools:
- VATIC
- Multimedia Annotation Tool (MAT)
- Anfi
- Praat
- AWS Transcribe
4. Crowdsourcing Platforms:
- Amazon Mechanical Turk
- Scale AI
- Appen
- CloudFactory
- Hive
These tools and platforms offer a range of features, including user-friendly interfaces, collaboration capabilities, quality control mechanisms, and integration with machine learning frameworks, enabling efficient and accurate data annotation at scale.
The Future: Trends and Innovations
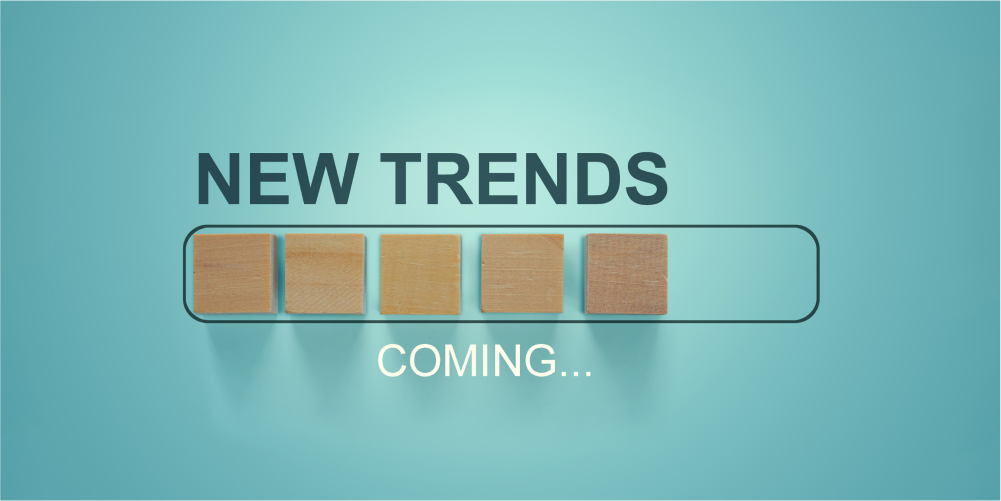
As AI technologies continue to evolve and find applications in ever-expanding domains, the role of data annotation is poised to become even more critical and several trends and innovations are shaping the future:
- Automated and Semi-Automated Annotation: With the advent of advanced machine learning techniques, such as transfer learning, active learning, and self-supervised learning, automated and semi-automated data annotation approaches are gaining traction. These methods leverage existing models and algorithms to pre-annotate data, reducing the need for manual annotation and accelerating the process while maintaining high accuracy.
- Crowdsourcing and Collective Intelligence: Crowdsourcing platforms and collective intelligence approaches are becoming increasingly popular, leveraging the power of distributed human intelligence to annotate large volumes of data efficiently and cost-effectively. These platforms often employ quality control mechanisms and incentive structures to ensure accurate and reliable annotations.
- Synthetic Data Generation: In scenarios where real-world data is scarce or challenging to obtain, synthetic data generation techniques are emerging as a viable solution. These methods leverage generative adversarial networks (GANs), computer graphics, and domain-specific simulations to create realistic, annotated synthetic data for training AI models.
- Annotation Marketplaces and Outsourcing: As the demand for high-quality annotated data continues to rise, annotation marketplaces and outsourcing services are gaining traction. These platforms connect businesses with experienced annotation providers, offering scalable and cost-effective solutions for large-scale data annotation projects.
- Domain-Specific Annotation Tools and Standards: With AI applications spanning diverse industries, the need for domain-specific annotation tools and standards is becoming increasingly apparent. These specialized tools and guidelines ensure accurate and consistent annotations tailored to specific use cases, such as medical imaging, autonomous driving, or financial risk analysis.
Unlock the Power of AI with Trantor
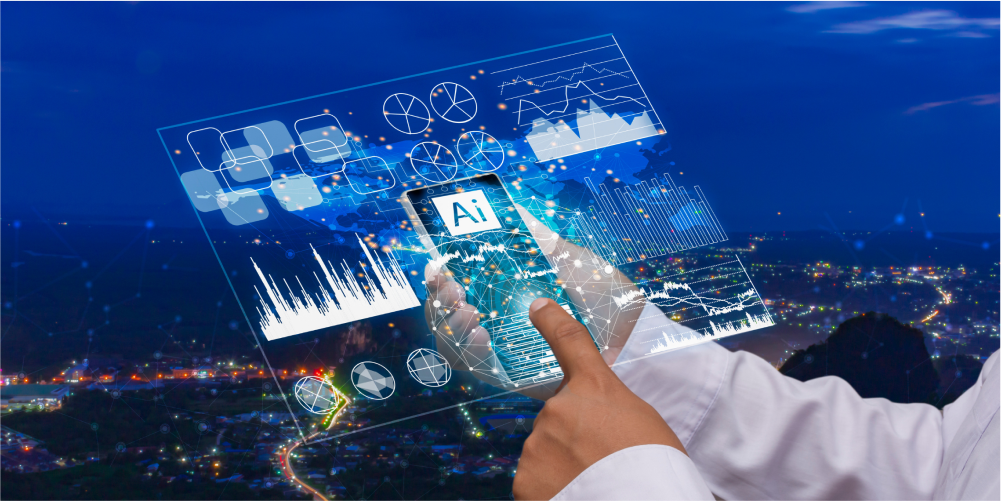
At Trantor, we understand the pivotal role data annotation plays in unlocking the true potential of AI and driving innovation across industries. Our team of experts specializes in providing comprehensive services, leveraging cutting-edge tools, methodologies, and industry best practices.
Our solutions are tailored to your specific needs, ensuring accurate, consistent, and high-quality annotations that fuel the development of reliable and trustworthy AI models. Whether you require image, text, video, audio, or sensor data annotation, our experienced annotators and domain experts work closely with you to understand your unique requirements and deliver annotated datasets that meet the highest standards.
By partnering with Trantor, you can accelerate your AI journey, overcome data annotation challenges, and empower your organization to harness the transformative power of AI. Elevate your AI initiatives with expert data annotation services and unlock a world of intelligent insights and innovative solutions.
Conclusion
In the era of AI-driven innovation, data annotation is the cornerstone of building intelligent systems that can revolutionize businesses and transform lives. By mastering the art, organizations can unlock the full potential of AI, gain a competitive edge, and drive sustainable growth.
At Trantor, we are committed to being your trusted partner in this journey, providing expert guidance, cutting-edge tools, and tailored solutions to navigate the complexities of modern technologies. Elevate your AI initiatives and embrace the future of intelligent technologies by embarking on your journey with us today.
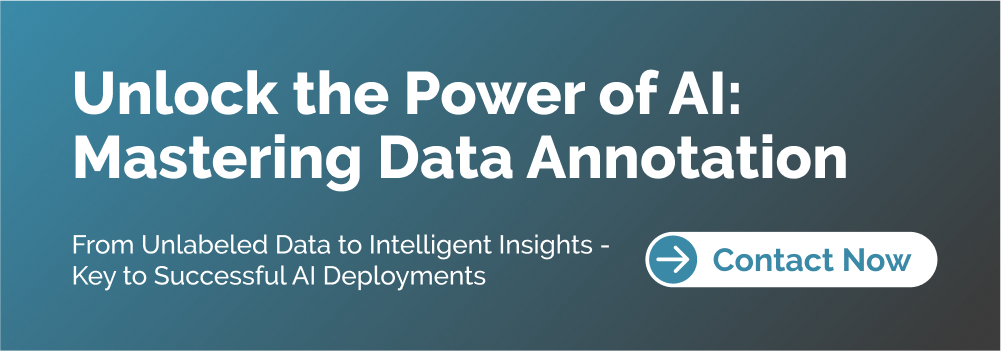