Artificial Intelligence, zBlog
Generative AI vs Predictive AI: All You Need to Know
Introduction
In the rapidly evolving landscape of artificial intelligence (AI), two distinct approaches have emerged: generative AI and predictive AI. While both are powerful tools, they serve different purposes and employ different techniques to achieve their respective goals. This blog post will delve into the intricacies of generative AI vs predictive AI, exploring their definitions, underlying methodologies, applications, and the key distinctions between them.
Understanding Generative AI
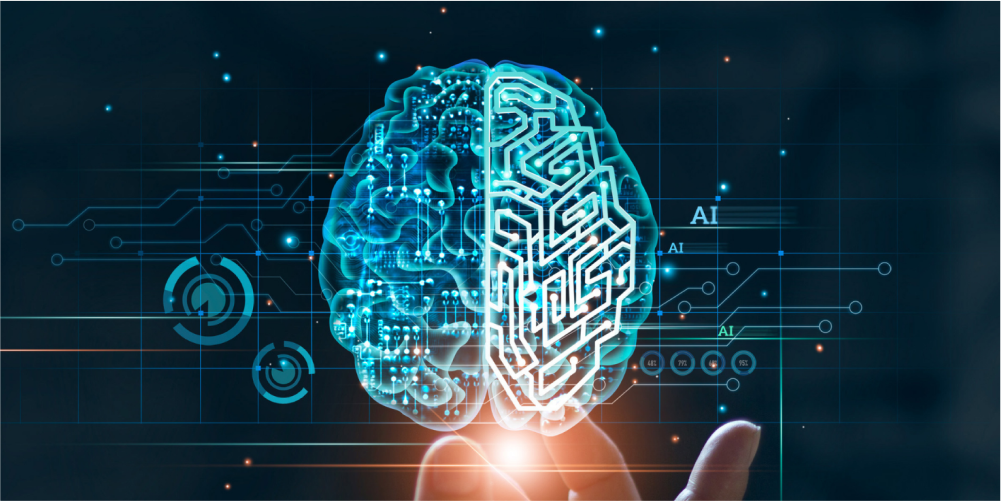
Generative AI, as the name suggests, is a branch of AI that focuses on generating new data, such as text, images, audio, or other forms of content, based on the patterns and relationships learned from existing data. This approach leverages deep learning techniques, particularly generative adversarial networks (GANs) and variational autoencoders (VAEs), to create entirely new and synthetic content that is similar to, but distinct from, the training data.
The fundamental principle behind generative AI is to learn the underlying probability distribution of the training data and then generate new samples that conform to that distribution. This process involves training a generative model on a large dataset, allowing it to capture the intricate patterns and relationships within the data.
Once the model is trained, it can generate new instances that share the characteristics of the training data but are unique and novel. This capability makes generative AI particularly valuable in various applications, including:
- Content Creation: Generative AI can be used to create realistic images, videos, music, and even written content, opening up new avenues for creativity and artistic expression.
- Data Augmentation: In machine learning, having a large and diverse dataset is crucial for training accurate models. Generative AI can be employed to augment existing datasets by generating synthetic data samples, improving model performance and robustness.
- Simulation and Synthetic Data Generation: Generative AI can create synthetic data for simulations, enabling the testing and evaluation of systems in controlled environments or scenarios where real-world data is scarce or difficult to obtain.
- Anomaly Detection: By learning the patterns of normal data, generative AI models can identify anomalies or outliers that deviate significantly from the expected distribution, making it useful for fraud detection, system monitoring, and predictive maintenance.
Understanding Predictive AI
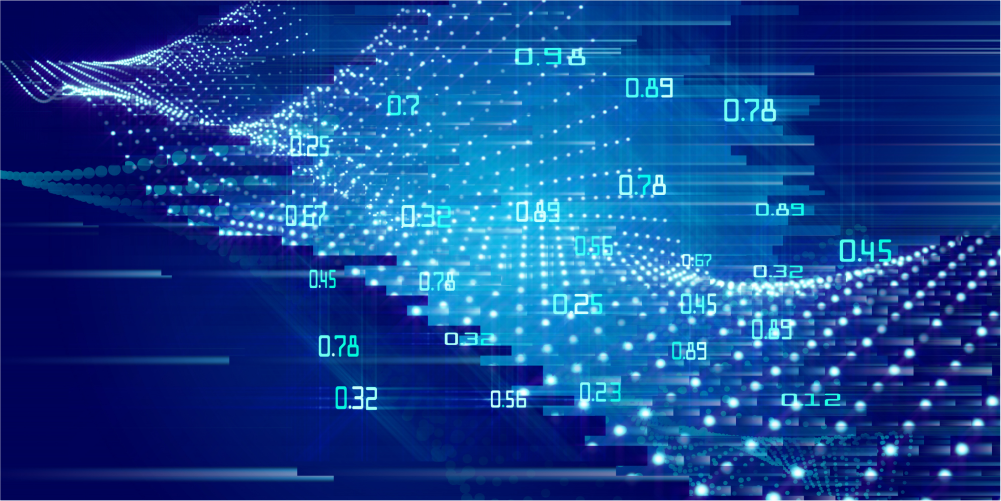
Predictive AI, on the other hand, is focused on making predictions or forecasts based on historical data and patterns. This approach leverages machine learning techniques, such as supervised learning algorithms like regression, classification, and time series analysis, to identify relationships and trends within the data.
The goal of predictive AI is to develop models that can accurately predict future outcomes or make informed decisions based on the patterns and relationships discovered in the training data. These models are trained on labeled data, where the desired output or target variable is known, allowing the model to learn the mappings between input features and the corresponding output.
Predictive AI finds applications in various domains, including:
- Forecasting and Trend Analysis: Predictive AI can be used to forecast future trends, sales, demand, or other variables based on historical data, enabling informed decision-making and strategic planning.
- Recommendation Systems: By analyzing user preferences, behavior, and other relevant data, predictive AI models can provide personalized recommendations for products, services, or content, enhancing user experience and driving engagement.
- Risk Assessment and Credit Scoring: Predictive AI models can assess the risk associated with lending or financial transactions by analyzing factors such as credit history, income, and other relevant data, enabling more informed and data-driven decision-making.
- Predictive Maintenance: By monitoring sensor data and analyzing patterns, predictive AI can anticipate equipment failures or maintenance needs, enabling proactive maintenance and reducing downtime.
- Healthcare and Disease Prediction: Predictive AI models can analyze patient data, including medical records, lab results, and other relevant information, to predict the likelihood of developing certain diseases or conditions, enabling early intervention and improved patient outcomes.
Key Differences: Generative AI vs Predictive AI
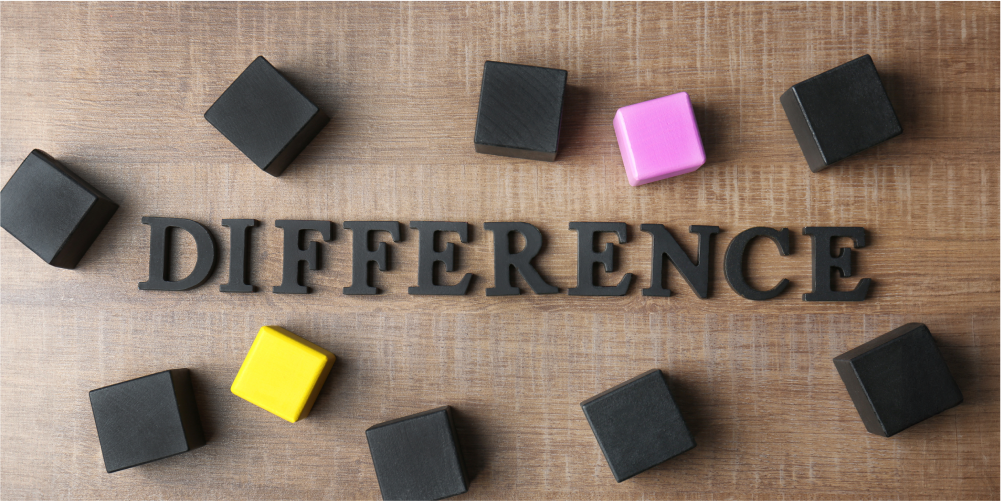
While both generative AI and predictive AI are powerful tools in the AI domain, they differ significantly in their approaches, methodologies, and applications. Here are some key distinctions between the two:
- Purpose: Generative AI aims to create new data or content based on learned patterns, while predictive AI focuses on making predictions or forecasts based on historical data.
- Techniques: Generative AI primarily employs deep learning techniques like GANs and VAEs, while predictive AI leverages supervised learning algorithms such as regression, classification, and time series analysis.
- Training Data: Generative AI models learn from unlabeled data, capturing the underlying probability distribution, while predictive AI models require labeled data with known outputs or target variables.
- Output: Generative AI produces new, synthetic data instances, whereas predictive AI provides predictions or forecasts based on input data.
- Applications: Generative AI is well-suited for content creation, data augmentation, and simulations, while predictive AI excels in forecasting, recommendation systems, risk assessment, and predictive maintenance.
- Evaluation Metrics: Generative AI models are often evaluated based on the quality and realism of the generated data, while predictive AI models are typically assessed based on their accuracy, precision, recall, or other relevant performance metrics.
It’s important to note that while generative AI and predictive AI have distinct approaches and applications, they can also be complementary in certain scenarios. For example, generative AI can be used to augment training data for predictive AI models, or predictive AI can be employed to guide the generation process in generative AI models.
Moreover, the rapid advancements in AI and the convergence of different techniques have led to the emergence of hybrid models that combine aspects of both generative and predictive AI. These hybrid approaches aim to leverage the strengths of both methodologies, enabling more sophisticated and versatile AI systems.
Harnessing the Power of AI
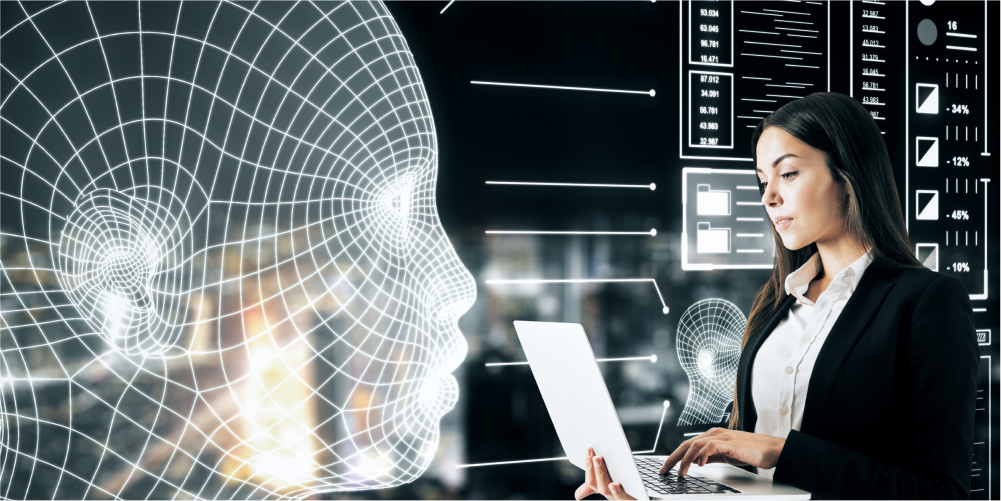
Trantor recognizes the immense potential of generative AI and predictive AI in driving innovation and solving complex challenges across various industries. With a team of highly skilled AI experts and a deep understanding of these cutting-edge technologies, Trantor is well-equipped to help organizations harness the power of AI to achieve their objectives.
Whether it’s generating synthetic data for training robust machine learning models, creating realistic content for enhanced user experiences, or developing accurate predictive models for informed decision-making, Trantor’s expertise in generative AI and predictive AI ensures tailored solutions that align with your specific needs.
By partnering with Trantor, you gain access to a wealth of knowledge and experience in AI, enabling you to stay ahead of the curve and capitalize on the transformative potential of these technologies. Reach out to Trantor today and embark on a journey of innovation, where the boundaries of what’s possible are continuously redefined.
Conclusion
In the ever-evolving realm of artificial intelligence, generative AI and predictive AI represent two distinct yet powerful approaches. Generative AI focuses on creating new data or content based on learned patterns, while predictive AI excels in making accurate predictions or forecasts based on historical data.
While their methodologies and applications may differ, both generative AI and predictive AI offer immense potential for innovation and problem-solving across various domains. By understanding the key distinctions between these two approaches, organizations can make informed decisions about which AI technique best aligns with their specific needs and objectives.
As AI continues to advance, the convergence of generative vs predictive AI techniques will likely lead to the development of even more sophisticated and versatile AI systems, pushing the boundaries of what’s possible. Trantor is at the forefront of these advancements, combining deep expertise in AI with a commitment to innovation and a passion for solving complex challenges.
By partnering with Trantor, organizations can harness the transformative power of AI, leveraging both generative and predictive techniques to drive business growth, enhance user experiences, and unlock new avenues for innovation. Reach out to Trantor today and embark on a journey where the possibilities are limitless, and the future is shaped by the convergence of cutting-edge AI technologies.
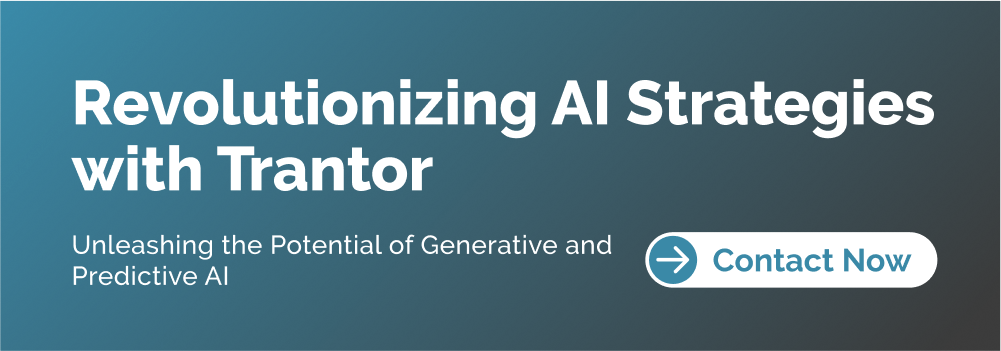